Descripción del título
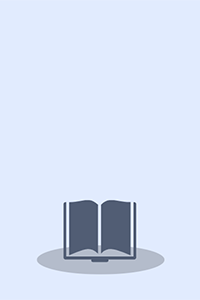
State space models have gained tremendous popularity in recent years in as disparate fields as engineering, economics, genetics and ecology. After a detailed introduction to general state space models, this book focuses on dynamic linear models, emphasizing their Bayesian analysis. Whenever possible it is shown how to compute estimates and forecasts in closed form; for more complex models, simulation techniques are used. A final chapter covers modern sequential Monte Carlo algorithms. The book illustrates all the fundamental steps needed to use dynamic linear models in practice, using R. Many detailed examples based on real data sets are provided to show how to set up a specific model, estimate its parameters, and use it for forecasting. All the code used in the book is available online. No prior knowledge of Bayesian statistics or time series analysis is required, although familiarity with basic statistics and R is assumed. Giovanni Petris is Associate Professor at the University of Arkansas. He has published many articles on time series analysis, Bayesian methods, and Monte Carlo techniques, and has served on National Science Foundation review panels. He regularly teaches courses on time series analysis at various universities in the US and in Italy. An active participant on the R mailing lists, he has developed and maintains a couple of contributed packages. Sonia Petrone is Associate Professor of Statistics at Bocconi University,Milano. She has published research papers in top journals in the areas of Bayesian inference, Bayesian nonparametrics, and latent variables models. She is interested in Bayesian nonparametric methods for dynamic systems and state space models and is an active member of the International Society of Bayesian Analysis. Patrizia Campagnoli received her PhD in Mathematical Statistics from the University of Pavia in 2002. She was Assistant Professor at the University of Milano-Bicocca and currently works for a financial software company
Monografía
monografia Rebiun23739005 https://catalogo.rebiun.org/rebiun/record/Rebiun23739005 m o d cr cn||||||||| 090824s2009 ne ob 000 0 eng d 2009926480 437009442 492091641 647818477 666990143 741649352 816325682 823122255 1005745380 1044280700 1097342842 1116713125 9780387772387 0387772383 9780387772370 0387772375 1282292153 ebk.) 9781282292154 ebk.) AU@ 000048715401 CDX 10800010 DEBSZ 396280676 NZ1 13117654 978-0-387-77237-0 Springer http://www.springerlink.com GW5XE eng pn GW5XE OCLCQ N$T OSU IDEBK OCLCQ HS0 OCLCQ OCLCF CDX E7B EBLCP MHW YDXCP DEBSZ OCLCQ Z5A OCLCQ VT2 CEF OCLCQ CNTRU S2H MAT 029000 bisacsh 519.502855133 22 SK 840 rvk ST 601 R01 rvk Petris, Giovanni Dynamic linear models with R Giovanni Petris, Sonia Petrone, Patrizia Campagnoli Dordrecht New York Springer-Verlag ©2009 Dordrecht New York Dordrecht New York Springer-Verlag 1 online resource 1 online resource Text txt rdacontent computer c rdamedia online resource cr rdacarrier Use R! Includes bibliographical references Introduction: basic notions about Bayesian inference -- Dynamic linear models -- Model specification -- Models with unknown parameters -- Sequential Monte Carlo methods State space models have gained tremendous popularity in recent years in as disparate fields as engineering, economics, genetics and ecology. After a detailed introduction to general state space models, this book focuses on dynamic linear models, emphasizing their Bayesian analysis. Whenever possible it is shown how to compute estimates and forecasts in closed form; for more complex models, simulation techniques are used. A final chapter covers modern sequential Monte Carlo algorithms. The book illustrates all the fundamental steps needed to use dynamic linear models in practice, using R. Many detailed examples based on real data sets are provided to show how to set up a specific model, estimate its parameters, and use it for forecasting. All the code used in the book is available online. No prior knowledge of Bayesian statistics or time series analysis is required, although familiarity with basic statistics and R is assumed. Giovanni Petris is Associate Professor at the University of Arkansas. He has published many articles on time series analysis, Bayesian methods, and Monte Carlo techniques, and has served on National Science Foundation review panels. He regularly teaches courses on time series analysis at various universities in the US and in Italy. An active participant on the R mailing lists, he has developed and maintains a couple of contributed packages. Sonia Petrone is Associate Professor of Statistics at Bocconi University,Milano. She has published research papers in top journals in the areas of Bayesian inference, Bayesian nonparametrics, and latent variables models. She is interested in Bayesian nonparametric methods for dynamic systems and state space models and is an active member of the International Society of Bayesian Analysis. Patrizia Campagnoli received her PhD in Mathematical Statistics from the University of Pavia in 2002. She was Assistant Professor at the University of Milano-Bicocca and currently works for a financial software company R (Computer program language) Linear models (Statistics) MATHEMATICS- Probability & Statistics- General. Linear models (Statistics) R (Computer program language) Bayes-Verfahren. Lineares dynamisches System. R (Programm) Electronic books Petrone, Sonia Campagnoli, Patrizia Print version Petris, Giovanni. Dynamic linear models With R. Dordrecht ; New York : Springer, ©2009 9780387772370 (OCoLC)310400804 Use R!