Descripción del título
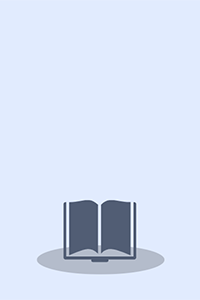
This book aims to present a viable alternative to the Hopfield Neural Network (HNN) model for analog computation. It is well known that the standard HNN suffers from problems of convergence to local minima, and requirement of a large number of neurons and synaptic weights. Therefore, improved solutions are needed. The non-linear synapse neural network (NoSyNN) is one such possibility and is discussed in detail in this book. This book also discusses the applications in computationally intensive tasks like graph coloring, ranking, and linear as well as quadratic programming. The material in the book is useful to students, researchers and academician working in the area of analog computation
Monografía
monografia Rebiun36326639 https://catalogo.rebiun.org/rebiun/record/Rebiun36326639 m o d | cr nn 008mamaa 130902s2014 ii s 00 0 eng d 9788132215639 9788132215646 9788132215622 9788132228967 10.1007/978-81-322-1563-9 doi UMA.RE eng UYQ bicssc COM004000 bisacsh 006.3 23 Ansari, Mohd. Samar. aut. http://id.loc.gov/vocabulary/relators/aut Non-Linear Feedback Neural Networks Recurso electrónico] VLSI Implementations and Applications by Mohd. Samar Ansari New Delhi Springer India 2014 New Delhi New Delhi Springer India New Delhi Springer India Imprint: Springer 2014 New Delhi New Delhi Springer India Imprint: Springer XXII, 201 p. 79 il XXII, 201 p. 79 il Text txt rdacontent computer c rdamedia online resource cr rdacarrier text file PDF rda Studies in Computational Intelligence 508 Bibliographic Level Mode of Issuance: Monograph Includes bibliographical references Introduction -- Background -- Voltage-mode Neural Network for the Solution of Linear Equations -- Mixed-mode Neural Circuit for Solving Linear Equations -- Non-Linear Feedback Neural Circuits for Linear and Quadratic Programming -- OTA-based Implementations of Mixed-mode Neural Circuits -- Appendix A: Mixed-mode Neural Network for Graph Colouring -- Appendix B: Mixed-mode Neural Network for Ranking This book aims to present a viable alternative to the Hopfield Neural Network (HNN) model for analog computation. It is well known that the standard HNN suffers from problems of convergence to local minima, and requirement of a large number of neurons and synaptic weights. Therefore, improved solutions are needed. The non-linear synapse neural network (NoSyNN) is one such possibility and is discussed in detail in this book. This book also discusses the applications in computationally intensive tasks like graph coloring, ranking, and linear as well as quadratic programming. The material in the book is useful to students, researchers and academician working in the area of analog computation English Engineering Systems engineering Electronics Computational Intelligence Circuits and Systems Mathematical Models of Cognitive Processes and Neural Networks Electronics and Microelectronics, Instrumentation Engineering Systems engineering Electronics Computational Intelligence Circuits and Systems Mathematical Models of Cognitive Processes and Neural Networks Electronics and Microelectronics, Instrumentation Studies in Computational Intelligence (CKB)1000000000238186 (DLC) (OCoLC) 1860-9503 81-322-1562-1 Studies in Computational Intelligence 508