Descripción del título
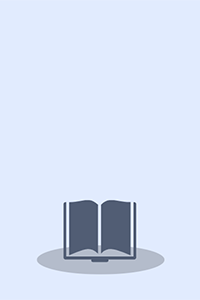
Machines capable of automatic pattern recognition have many fascinating uses in science and engineering as well as in our daily lives. Algorithms for supervised classification, where one infers a decision boundary from a set of training examples, are at the core of this capability. Tremendous progress has been made in refining such algorithms; yet, automatic learning in many simple tasks in daily life still appears to be far from reach. This book takes a close view of data complexity and its role in shaping the theories and techniques in different disciplines and asks: What is missing from current classification techniques? When the automatic classifiers are not perfect, is it a deficiency of the algorithms by design, or is it a difficulty intrinsic to the classification task? How do we know whether we have exploited to the fullest extent the knowledge embedded in the training data? Data Complexity in Pattern Recognition is unique in its comprehensive coverage and multidisciplinary approach from various methodological and practical perspectives. Researchers and practitioners alike will find this book an insightful reference to learn about the current status of available techniques as well as application areas
Monografía
monografia Rebiun21577133 https://catalogo.rebiun.org/rebiun/record/Rebiun21577133 m o d cr cn||||||||| 080226s2006 enka ob 001 0 eng d 122930594 154690404 288148013 320966658 401431901 516040379 613463626 647650378 756423520 880095764 987639169 994766910 1005749453 1035707903 1044270610 1056315567 1058368928 1060827853 1075542153 1078212180 9781846281723 1846281725 9781846281716 1846281717 hbk.) 9786611067540 661106754X 10.1007/978-1-84628-172-3 doi 978-1-84628-171-6 Springer http://www.springerlink.com GW5XE eng pn GW5XE EBLCP N$T YDXCP CEF NUI CNTRU YNG AZU E7B OCLCQ IDEBK OCLCO OCLCQ A7U OCLCQ OCLCF BEDGE OCLCQ SLY DEBSZ OCLCQ UAB Z5A OTZ OCLCQ ESU OCLCQ VT2 BUF STF OCLCQ U3W AU@ OCLCQ WYU ICG YOU CANPU COM 047000 bisacsh Data complexity in pattern recognition Mitra Basu and Tin Kam Ho (eds) London Springer ©2006 London London Springer 1 online resource (xv, 300 pages) illustrations 1 online resource (xv, 300 pages) Text txt rdacontent computer c rdamedia online resource cr rdacarrier text file PDF rda Advanced information and knowledge processing Includes bibliographical references and index Theory and Methodology -- Measures of Geometrical Complexity in Classification Problems -- Object Representation, Sample Size, and Data Set Complexity -- Measures of Data and Classifier Complexity and the Training Sample Size -- Linear Separability in Descent Procedures for Linear Classifiers -- Data Complexity, Margin-Based Learning, and Popper's Philosophy of Inductive Learning -- Data Complexity and Evolutionary Learning -- Classifier Domains of Competence in Data Complexity Space -- Data Complexity Issues in Grammatical Inference -- Applications -- Simple Statistics for Complex Feature Spaces -- Polynomial Time Complexity Graph Distance Computation for Web Content Mining -- Data Complexity in Clustering Analysis of Gene Microarray Expression Profiles -- Complexity of Magnetic Resonance Spectrum Classification -- Data Complexity in Tropical Cyclone Positioning and Classification -- Human-Computer Interaction for Complex Pattern Recognition Problems -- Complex Image Recognition and Web Security Machines capable of automatic pattern recognition have many fascinating uses in science and engineering as well as in our daily lives. Algorithms for supervised classification, where one infers a decision boundary from a set of training examples, are at the core of this capability. Tremendous progress has been made in refining such algorithms; yet, automatic learning in many simple tasks in daily life still appears to be far from reach. This book takes a close view of data complexity and its role in shaping the theories and techniques in different disciplines and asks: What is missing from current classification techniques? When the automatic classifiers are not perfect, is it a deficiency of the algorithms by design, or is it a difficulty intrinsic to the classification task? How do we know whether we have exploited to the fullest extent the knowledge embedded in the training data? Data Complexity in Pattern Recognition is unique in its comprehensive coverage and multidisciplinary approach from various methodological and practical perspectives. Researchers and practitioners alike will find this book an insightful reference to learn about the current status of available techniques as well as application areas English Pattern recognition systems Pattern perception Classification Computational complexity Reconnaissance des formes (Informatique) Perception des structures Complexité de calcul (Informatique) COMPUTERS- Optical Data Processing. Pattern perception. Classification. Computational complexity. Reconnaissance des formes (informatique) . Perception des structures. Complexité de calcul (Informatique) . Pattern recognition systems. Informatique. Classification. Computational complexity. Pattern perception. Pattern recognition systems. Electronic books Basu, Mitra Ho, Tin Kam Print version Data complexity in pattern recognition. London : Springer, ©2006 1846281717 9781846281716 (DLC) 2005936159 (OCoLC)62761587 Advanced information and knowledge processing